Artificial Intelligence (AI) continues to transform various industries, driving innovation, efficiency, and new functionalities. The evolution of AI models has been rapid, with each new release pushing the boundaries of what technology can achieve. This article delves into the latest AI models released, exploring their advancements, functionalities, and the broader impact these innovations are having on AI technology. We will examine relevant examples, case studies, and statistics to provide a comprehensive understanding of the current landscape.
1. Introduction to AI Advancements
The Importance of AI in Modern Technology
AI has become an integral part of modern technology, underpinning advancements in various sectors such as healthcare, finance, retail, and transportation. The ability of AI to learn, adapt, and improve over time makes it a powerful tool for solving complex problems and enhancing operational efficiency.
Key drivers of AI advancements include:
- Increased computational power and data availability.
- Developments in machine learning algorithms and techniques.
- Growing investments in AI research and development.
2. Overview of New AI Models
OpenAI’s GPT-4
One of the most anticipated AI models of 2024 is OpenAI’s GPT-4, an advanced language model designed to understand and generate human-like text. Building on the success of its predecessor, GPT-3, this new model boasts numerous enhancements:
- Improved Language Understanding: GPT-4 has a deeper comprehension of context and nuance, resulting in more accurate and coherent responses.
- Increased Parameters: With over 175 billion parameters, GPT-4 offers superior performance in natural language processing (NLP) tasks.
- Advanced Natural Language Generation: The model can generate high-quality text for a variety of applications, including content creation, customer support, and conversational agents.
Google’s MUM (Multitask Unified Model)
Google’s MUM is another groundbreaking AI model that aims to enhance search and information retrieval. MUM’s capabilities include:
- Multimodal Understanding: MUM can process text, images, and other modalities simultaneously, providing richer and more informative search results.
- Language Generalisation: The model can understand and generate content in multiple languages, enhancing global accessibility.
- Complex Task Assistance: MUM can assist users with complex tasks, such as planning trips or researching intricate topics, by aggregating and synthesising relevant information from various sources.
DeepMind’s AlphaFold 2
DeepMind’s AlphaFold 2 represents a significant leap in AI-driven protein folding, a critical aspect of biological research. Key advancements include:
- High-Accuracy Predictions: AlphaFold 2 achieves state-of-the-art accuracy in predicting protein structures, aiding in drug discovery and biological research.
- Expedited Research: The model dramatically accelerates the process of protein structure determination, which traditionally took months or years.
- Open Access and Community Impact: DeepMind has made AlphaFold 2’s predictions and code publicly available, fostering collaboration and innovation within the scientific community.
3. Case Studies and Applications
Healthcare: Revolutionising Diagnosis and Treatment
AI models are revolutionising the healthcare industry by enhancing diagnostic accuracy and personalising treatment plans. Key examples include:
- AI-Powered Diagnostics: AI models like Google’s DeepMind Health can analyse medical images, such as X-rays and MRIs, with high accuracy, aiding doctors in early detection of diseases.
- Personalised Medicine: AI-driven genomics models can analyse genetic data to deliver personalised treatment plans based on individual patient profiles.
- Virtual Health Assistants: AI-powered virtual assistants provide patients with 24/7 access to healthcare advice, reducing the burden on medical professionals and improving patient outcomes.
Finance: Enhancing Fraud Detection and Risk Management
The finance sector has embraced AI to improve fraud detection, risk management, and customer experiences. Notable applications include:
- Fraud Detection: AI models can analyse transaction patterns and identify anomalous behaviour, enabling real-time detection and prevention of fraudulent activities.
- Risk Management: AI-driven analytics assess risk factors and predict market trends, helping financial institutions to make informed investment decisions.
- Customer Experience: AI-powered chatbots and virtual assistants streamline customer support, providing personalised assistance and enhancing satisfaction.
Retail: Transforming Customer Experiences
In the retail industry, AI models are transforming customer experiences by personalising recommendations, optimising inventory management, and improving customer service. Key examples include:
- Personalised Recommendations: AI-driven recommendation engines analyse customer preferences and purchase history to deliver tailored product suggestions, increasing engagement and sales.
- Inventory Optimisation: AI models predict demand patterns and optimise inventory levels, reducing stockouts and minimising excess inventory.
- Customer Service: AI-powered chatbots handle customer inquiries efficiently, providing instant responses and freeing up human agents for more complex tasks.
4. Emerging Trends in AI Technology
Explainable AI (XAI)
As AI models become more complex and pervasive, the need for explainability and transparency has grown. Explainable AI (XAI) aims to make AI decisions understandable to humans:
- Interpretability: XAI techniques provide insights into how AI models reach their predictions, enhancing trust and accountability.
- Regulatory Compliance: XAI assists organisations in meeting regulatory requirements by demonstrating the rationale behind AI-driven decisions.
- Ethical AI: Explainability promotes ethical AI usage by identifying and mitigating biases and ensuring fairness in decision-making processes.
Federated Learning
Federated learning is a distributed approach to training AI models that preserves data privacy. It allows models to learn from decentralised data sources without compromising individual privacy:
- Data Privacy: Federated learning protects sensitive data by training models locally on user devices, without transferring raw data to central servers.
- Scalability: The approach enables AI training on vast and diverse datasets distributed across multiple locations, improving model accuracy and generalisation.
- Collaboration: Federated learning facilitates collaboration among organisations, allowing them to benefit from shared insights without revealing proprietary data.
AI Ethics and Governance
As AI’s influence grows, ethical considerations and governance frameworks have become critical to ensuring responsible AI development and deployment:
- Fairness and Bias Mitigation: Organisations are implementing measures to identify and reduce biases in AI models, ensuring fair and unbiased outcomes.
- AI Governance: Establishing governance frameworks that define ethical guidelines, accountability mechanisms, and compliance standards for AI development.
- Public Awareness: Educating the public about AI technologies, their benefits, and potential risks to foster informed decision-making and societal trust.
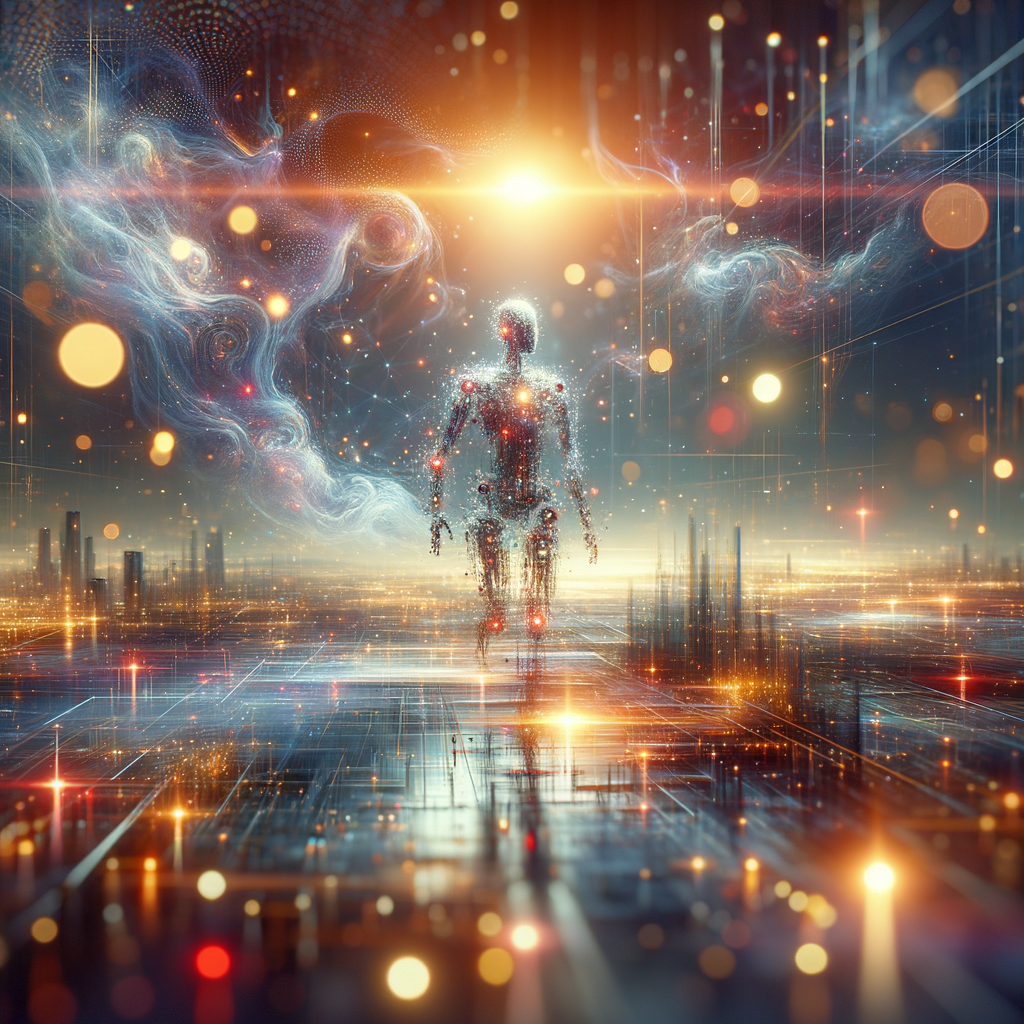
5. Impact of AI Advancements on Various Industries
Healthcare
AI advancements are revolutionising healthcare by enhancing diagnostic accuracy, personalising treatment plans, and improving patient outcomes:
- Early Disease Detection: AI models can analyse medical images and genetic data to detect diseases at early stages, improving prognosis and survival rates.
- Personalised Medicine: AI-driven genomics models tailor treatments based on individual patient profiles, maximising therapeutic efficacy.
- Operational Efficiency: AI streamlines administrative tasks, such as appointment scheduling and medical record management, allowing healthcare professionals to focus on patient care.
Finance
The finance sector is leveraging AI to enhance fraud detection, risk management, and customer experiences:
- Real-Time Fraud Detection: AI algorithms identify and prevent fraudulent activities by analysing transaction patterns in real time.
- Predictive Analytics: AI models predict market trends and assess risk factors, enabling informed investment decisions and portfolio management.
- Customer Experience: AI-powered chatbots and virtual assistants provide personalised support, enhancing customer satisfaction and loyalty.
Retail
AI is transforming the retail industry by personalising customer experiences and optimising supply chain operations:
- Enhanced Product Recommendations: AI models analyse customer preferences to deliver personalised product suggestions, boosting sales and engagement.
- Inventory Management: AI algorithms optimise inventory levels based on demand forecasts, reducing stockouts and excess inventory.
- Customer Service Automation: AI-powered chatbots handle routine inquiries, improving response times and freeing human agents for complex tasks.
Manufacturing
AI advancements are driving innovation in the manufacturing sector by enhancing production processes and ensuring quality control:
- Predictive Maintenance: AI models predict equipment failures and schedule maintenance, minimising downtime and reducing costs.
- Quality Assurance: AI-powered inspection systems detect defects in real time, ensuring high-quality products and reducing waste.
- Optimised Production: AI algorithms optimise production schedules and resource allocation, improving efficiency and productivity.
6. Challenges and Future Directions in AI
Addressing AI Bias
One of the main challenges in AI development is addressing bias within models, which can lead to unfair outcomes:
- Bias Detection Tools: Developing tools to identify and measure biases in AI models.
- Dataset Diversity: Ensuring training datasets are diverse and representative to minimise bias.
- Transparent Algorithms: Implementing transparent algorithms that allow for the examination of AI decision-making processes.
AI Regulation and Compliance
With AI technology rapidly advancing, ensuring compliance with ethical guidelines and regulatory standards is paramount:
- Establishing Standards: Developing global standards and guidelines for AI development and deployment.
- Monitoring and Auditing: Implementing mechanisms for the ongoing monitoring and auditing of AI systems for compliance with ethical standards.
- Public Policy: Collaborating with policymakers to develop laws and regulations that govern AI use and ensure accountability.
Future Directions in AI
AI technology is continually evolving, and several emerging areas hold promise for the future, including:
- AI in Edge Computing: Bringing AI processing closer to data sources to reduce latency and improve real-time decision-making.
- AI-Driven Cybersecurity: Enhancing cybersecurity measures with AI to detect and respond to threats more efficiently.
- Human-AI Collaboration: Developing systems that enable seamless collaboration between human workers and AI, augmenting human capabilities.
Conclusion: Key Takeaways
The release of new AI models in 2024 marks a significant step forward in the field of artificial intelligence, bringing about innovative functionalities and technological advancements. The key takeaways from this exploration include:
- AI Advancements: The new AI models, such as OpenAI’s GPT-4, Google’s MUM, and DeepMind’s AlphaFold 2, showcase substantial improvements in language understanding, information retrieval, and scientific research.
- Applications Across Sectors: AI is revolutionising various industries, including healthcare, finance, retail, and manufacturing, by enhancing efficiency, personalisation, and operational excellence.
- Emerging Trends: Trends such as Explainable AI, federated learning, and AI ethics and governance highlight the evolving nature of AI technology and the importance of responsible and transparent AI development.
- Challenges and Future Directions: Addressing AI bias, ensuring regulatory compliance, and exploring future directions, such as AI in edge computing and human-AI collaboration, will be critical for the continued advancement of AI technology.
As AI technology progresses, staying informed about the latest developments and understanding their implications will be paramount for businesses, researchers, and policymakers. By embracing these advancements and addressing the associated challenges, we can harness the full potential of AI to drive innovation, solve complex problems, and create a better future.